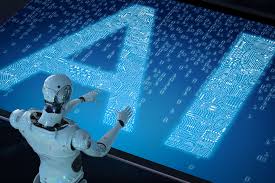
Meteorologists have been using computer generated weather forecasts since 1955. Massive computers used complex algorithms to make these predictions. Today, supercomputers are able to use amassed data from global weather stations, satellites, and virtually all of the observable data that the national weather service collects to make more accurate overall predictions. Yet, for all this data and analysis, the forecasts are not perfect. Why do we still get caught in the rain on days when sunny skies were predicted? The answer is as simple as it is complex. The collected and considered data show an incomplete picture. Weather patterns are highly volatile and affected by minor alterations in data. They are the perfect illustration of chaos theory. Is there any actual harm in getting caught in the rain without an umbrella tomorrow because the computer got it wrong? Not really, but what if the computer’s prediction was about something far more important?
The old computer algorithms are now being replaced by more complex computing systems with virtually limitless data to consider. These new systems are called artificial intelligence or are said to have machine learning. These systems can produce data in the blink of an eye but do not have the actual ability to analyze all the nuances of that data in the manner of a human being. Developers, with great hubris, pass off this computing as artificial intelligence because, in doing so, they can capitalize on our desire to reach into the future, realize scientific achievement, and save money on production. In her writings on the fallacies of artificial intelligence, Melanie Davis states, “AI is harder than we think because we are largely unconscious of the complexity of our own thought processes” (Davis, 2021). The subtle nuance of human thought has yet to be replicated. Despite many critics asking for a pause, businesses are rapidly adopting AI.
Healthcare is one of the primary industries now utilizing this so-called AI technology. Specifically, AI technology is currently in use for glycemic control for diabetics, diagnostic imaging, EKG analysis, fetal monitor strip analysis, inpatient falls prediction, lab sample analysis, and readmission prediction. Many of these uses have been in place for decades in some form, and many more are now emerging.
The computerized analysis performed by an EKG machine is an algorithm-based evaluation that has been in use for decades. This analysis is not always accurate for different reasons. As we know, EKGs are used to detect cardiac issues. Trained personnel connect the patient to the machine and these machines then plot a cardiac rhythm and determine a cardiac condition based on its input. A doctor then overreads this EKG and either agrees or disagrees with the machine’s determination. If the machine gets it wrong in this setting, the MD is there to verify. Unfortunately, this level of verification is not universal.
Computerized falls prediction is a popular AI element in healthcare where the electronic medical record (EMR) system uses patient data such as medical history, age, current medications, etc., to predict whether a patient is a low or high fall risk, and to prompt fall precautions to be initiated. These fall predictors have often been found to be inaccurate based on patient history or condition. Nursing oversight of this machine tool is more relaxed than the physician oversight of EKGs. Poor predictions are often not changed, leaving patients to suffer from insufficient precautions. The nuances of a patient’s condition require strict oversight and implementation for the patient’s safety.
Fetal monitoring strip analysis is yet another AI tool that has had issues with reliability. The GE Centricity Perinatal system analyzes and records fetal monitoring strips for laboring mothers. GE bills its system as “a second set of eyes when you need it the most”. GE further states that “in the labor and delivery room, decisions need to be made quickly” (GE, 2023). Though there is value in the ability to analyze information quickly, accuracy is far more important than speed. Over the last ten years, GE has had multiple issues with accuracy with its Centricity Perinatal system, including assigning the incorrect analyzed data to the proper patient.
The WHO (World Health Organization) is warning that the meteoric rise of AI tools in healthcare threatens the safety of patients if caution is not exercised. Precipitous adoption of untested systems could lead to errors by healthcare workers, cause harm to patients, erode trust in AI, and undermine the potential long-term benefits and uses of such technologies around the world (Kelly, 2023).
Who bears the blame when errors occur with AI in healthcare and a patient suffers? Is it the AI manufacturer, the healthcare system, or care personnel like doctors and nurses? This answer is not easy, as the fault could be with any or all of the above. Understanding the healthcare process is necessary to help unravel this puzzle.
Hospital systems have a duty to ensure the safety of patients. A journal article from 2021 states that, it is the belief of some legal experts that Negligent Credentialing Theories that hold health systems or physician groups liable for not properly vetting a physician who deviates from the standard of care could be applied to hospital systems that insufficiently vet an AI/ML system prior to clinical implementation (George Maliha S. G., 2021). This is in addition to the vicarious liability that hospital systems have for the errors of their employees.
Healthcare providers can also be at fault for AIrelated errors that cause injury. An increasing issue with these so-called AI systems is that they are being used not as a “second set of eyes,” as GE states in their marketing material for Centricity Perinatal, but rather as the first and sometimes only set of eyes. Many nurses in hospitals that use fall predictors are failing to do an independent fall risk analysis of the patients and are solely relying on the fall risk predictors which places the person at risk. Some obstetricians and labor and delivery nurses rely solely on programs like Centricity Perinatal to analyze and interpret fetal monitoring, and some radiologists are overly reliant on AI to spot anomalies in imaging.
Just as AI can predict outcomes in human health, real human intelligence can predict outcomes in AI. An article earlier this year made the following prediction: “AI systems are prone to mistakes, which might cause serious issues for patients…. There is a good risk that an AI-related mistake will have a long-term impact on a patient’s life”. The same article coined a new term, the AI chasm, the lack of accuracy in machine learning studies, which frequently results in erroneous predictions (Tasseef Ayoub Shaikh, 2023). Another article posed, “With increasing integration of artificial intelligence and machine learning in medicine, there are concerns that algorithm inaccuracy could lead to patient injury and medical liability” (George Maliha S. G., 2021, p. 17). The discussion about accuracy has many wondering how healthcare providers view these concerns in the rapidly developing use of AI in treating patients.
In a recent study from January 2023, a cohort of physicians and medical students were asked questions to determine providers’ readiness for artificial intelligence tools in the clinical setting. In one question to the 82 physicians and 211 medical students, 36.77 % of participants believe physicians making the final decision should be legally liable for any medical error when a physician uses AI-based decision support (adhari AlZaabi, 2023). This means 63% of the physicians polled do not believe they should be held accountable for their erroneous decisions based on artificial intelligence guidance. This is undoubtedly discomforting to the patients who suffered an injury and future patients that someday could. The introduction of AI into healthcare has created a complicated tapestry of data, assessment, analysis, intervention, and results. The levels and types of use are corporate driven decisions. Healthcare systems claim the move to AI is about increased patient access, and improved care, but cost reduction and profit realization are unspoken goals for this move and this element, though downplayed by healthcare organizations, cannot be ignored. AI is being introduced to eliminate overheads and make forensic records analysis even more difficult. The obfuscation even extends to AIrecorded data being entered into the medical record as if a human being had entered it.
Screening potential medical malpractices cases is becoming more challenging. Convoluted medical records become increasingly more difficult to follow and analyze. Big damage cases are often “red herrings” without all four pillars necessary to bring a suitable resolution for the plaintiff. The interweave of system driven data conclusions and provider-initiated response blurs the lines of responsibility for errors and damages and defense teams use this to full advantage. To operate efficiently plaintiff firms, have to be equally adept at cost effective medical case analysis as with the pursuit of justice through the legal system. This is where firms need to be more adroit in their preparation.
ADROIT® specializes in cutting dead-end threads and unraveling the convoluted weavings that healthcare systems and their EMRs use to hide errors that cause injury. We handle the medicine so attorneys can focus on the law and on obtaining justice for clients that have been injured. We use real human intelligence backed by years of experience. For this, there is no AI substitute.
This article appears in the June 2024 Edition of the SCAJ Justice Bulletin. The entire online publication can be found here. https://www.scaj.com/?pg=NewBulletin